The massive growth of live streaming, especially gaming-focused content, has led to an overall increase in global bandwidth consumption. Certain services see their quality diminished at times of peak consumption, degrading the quality of the content. This trend generates new research related to optimizing image quality according to network and service conditions. In this work we present a gaming streaming use case optimization on a real multisite 5G environment. The paper outlines the virtualized workflow of the use case and provides a detailed description of the applications and resources deployed for the simulation. This simulation tests the optimization of the service based on the addition of Artificial Intelligence (AI) algorithms, assuring the delivery of content with good Quality of Experience (QoE) under different working conditions. The AI introduced is based on Deep Reinforcement Learning (DRL) algorithms that can adapt, in a flexible way, to the different conditions that the multimedia workflow could face. That is, adapt, through corrective actions, the streaming bitrate, in order to optimize the QoE of the content on a real-time multisite scenario. The results of this work demonstrate how we have been able to minimize content losses, as well as the fact of obtaining high audiovisual multimedia quality results with higher bitrates, compared to a service without an optimizer integrated in the system. In a multi-site environment, we have achieved an improvement of 20 percentage points in terms of blockiness efficiency and also 15 percentage points in block loss.
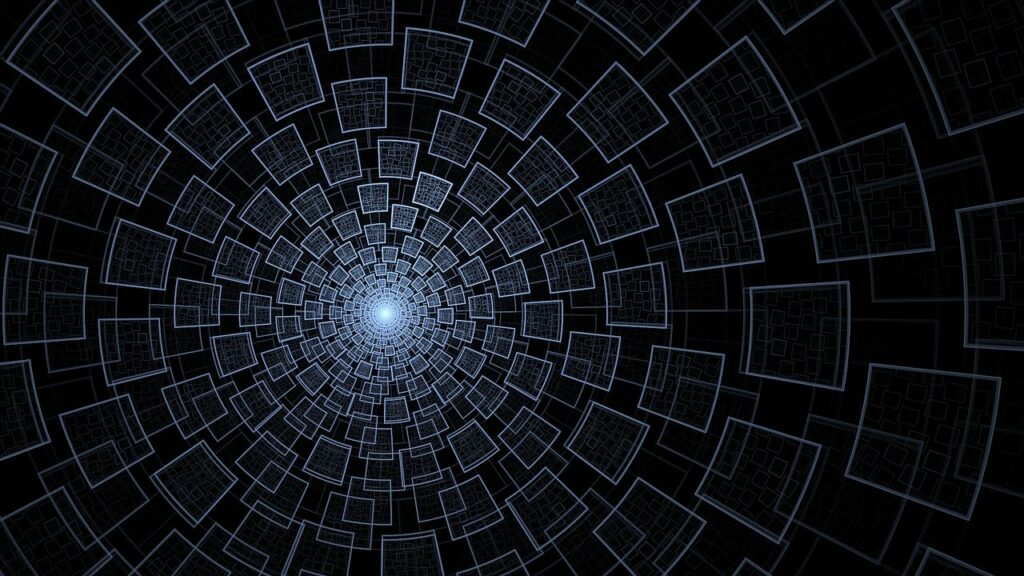